Lab Members
Home » David Beniaguev
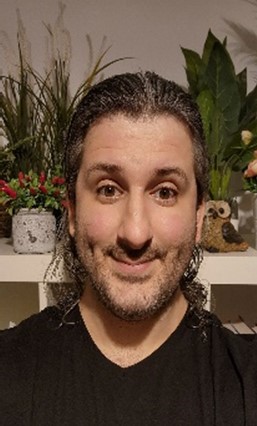
David Beniaguev
Post Doc
Single biological neurons as temporally-precise spatio-temporal pattern recognizers. Trying to understand how single neurons perform computations, in particular, how dendrites allow neurons to perform complex spatial computations and whether they also allow the neuron to utilize the temporal dimension as an additional axis for packing even more useful computations inside a single neuron.