ELSC Heller Lecture Series
Home » Heller Lecture Series » Deep language models as a cognitive model for natural language processing in the human brain
Heller Lecture Series in Computational Neuroscience
Dr. Uri Hasson
Princeton University
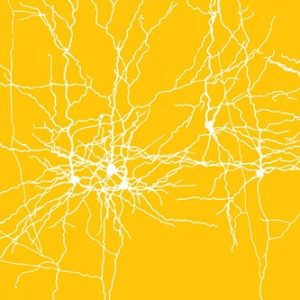
On the topic of:
Deep language models as a cognitive model for natural language processing in the human brain
Naturalistic experimental paradigms in neuroimaging arose from a pressure to test the validity of models we derive from highly controlled experiments in real-world contexts. In many cases, however, such efforts led to the realization that models developed under particular experimental manipulations failed to capture much variance outside the context of that manipulation. Recent advances in artificial neural networks provide an alternative computational framework to model cognition in natural contexts. In contrast to the simplified and interpretable hypotheses we test in the lab, these models do not learn simple, human-interpretable rules or representations of the world. Instead, they use local computations to interpolate over task-relevant manifolds in high-dimensional parameter space. Counterintuitively, over-parameterized deep neural models are parsimonious and straightforward, as they provide a versatile, robust solution for learning diverse functions in natural contexts. Naturalistic paradigms should not be deployed as an afterthought if we hope to build models of the brain and behavior that extend beyond the laboratory into the real world.
In this talk, I will use language as an example to contrast the two perspectives. Traditionally, the investigation of the neural basis of language relied on classical language models, which use explicit symbolic representations of parts of speech (like nouns, verbs, adjectives, adverbs, and determiners) combined with rule-based logical operations embedded in hierarchical tree structures (Chomsky 1986). Recently, advances in deep learning have led to the development of a new family of deep language models (DLMs), which were remarkably successful in many natural language processing (NLP) tasks in the wild. From a linguist’s perspective, the applied success of deep language models (DLMs) is striking because they rely on a very different cognitive architecture than the classical models: 1) DLMs do not parse words into parts of speech but rather encode words as vectors (sequences of real numbers) termed embeddings and rely on a series of simple arithmetic operations (as opposed to explicit rules) to generate the desired linguistic output; 2) embeddings are learned from real-world textual examples, by seeing how language is being used in the wild, without being endowed with any prior knowledge about the structure of language; 3) word embeddings are sensitive to the structural (grammatical) and semantic relationships between words in the text.; 4) learning is guided by simple objectives, such as maximizing the ability to predict the next word in a string of sentences. In the talk, I will argue that biological and artificial neural networks code language similarly by relying on context-based embeddings (vectors). Furthermore, I will present evidence that similar to deep language models, the human brain has the capacity to predict the content of the upcoming word before it has been articulated, an objective that was found highly effective for training such models.
Recorded Heller lectures are available online on the ELSC YouTube channel.
When
Where