ELSC Seminar Series
Home » ELSC Seminar Series » Charting the space of solutions for recurrent neural networks
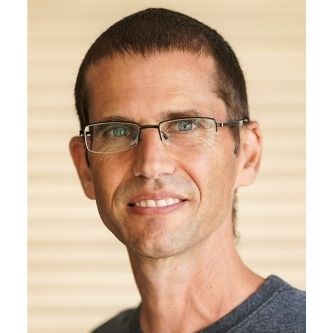
Prof. Omri Barak
Charting the space of solutions for recurrent neural networks
In recent years Recurrent Neural Networks (RNNs) were successfully used to model the way neural activity drives task-related behavior in animals, operating under the implicit assumption that the obtained solutions are universal. Observations in both neuroscience and in machine learning challenge this assumption. Animals can approach a given task with a variety of strategies, and training machine learning algorithms introduces the phenomenon of underspecification. These observations imply that every task is associated with a space of solutions. To date, the structure of this space is not understood, limiting the approach of comparing RNNs with neural data.Here, we characterize the space of solutions associated with a given task, and how hyperparameters bias networks within this space. We first study a simple two-neuron network on a task that leads to multiple solutions. We trace the nature of the final solution back to the network’s initial connectivity, and identify discrete dynamical regimes that underly this diversity. We then examine several neuroscience-inspired tasks. We find a rich set of solutions, even under identical hyperparameters. We uncover this variety by testing the trained networks’ ability to extrapolate, as a perturbation to a system often reveals hidden structure. Furthermore, we relate extrapolation patterns to specific dynamical objects and to effective algorithms found by the networks. Taken together, our results shed light on the concept of the space of solutions and its uses both in Machine learning and in Neuroscience.
Seminar Date & Time:
Notifications are sent to ELSC seminar mailing list, subscribe here.