If you ask a neuroscientist to draw a neural network, the resulting sketch most probably will look like the picture on the left, which was drawn in 1899 by the great neuroanatomist and Nobel Laureate Santiago Ramon y Cajal. However, if you ask a computer scientist to do the same, the result most probably will look like the diagram on the right.


You may then find yourself asking: “Do these two researchers speak the same language?” Well, the answer to that question is no. But a recent article, published in the prestigious journal Neuron by David Beniaguev and colleagues, shows how these two fields of research might talk more closely to (and inspire) each other.
In this article, researchers from the labs of Idan Segev and Mickey London found a systematic way to transform the detailed structure of single neurons into an equivalent deep neural network (DNN). These DNNs mimic the input/output relationship of a single cortical neuron with great precision. By doing this, the researchers present an elegant way to translate any biological neuron into the language of artificial neural networks so that these two entities can now be directly compared.
The DNN representation also provides new biophysical insights about the synaptic input conditions that can or cannot generate an output spike. Additionally, the researchers propose a new type of DNN architecture whereby the artificial neuron is replaced by an already deep unit, which represents biological neurons more accurately. With this new architecture, future researchers will find it easier to integrate new knowledge into the next generation of DNNs.
Beniaguev et al. further asked, what size artificial neural network is needed in order to match the activity of one biological neuron? They found that simulating a single cortical neuron required around 600-900 of the classical artificial “point neurons.” Their finding illustrates the potential computational complexity available to a single biological neuron, and future work will determine what aspects of these computational capabilities are actually employed by the brain.
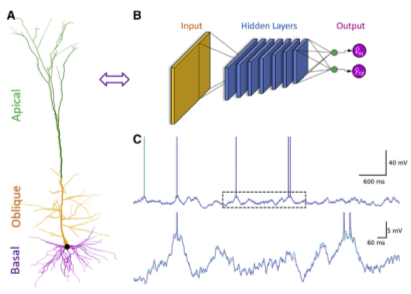
Figure 1: (A) A detailed model of layer 5 cortical pyramidal cell. (B) The equivalent deep neural network for this neuron. (C) Top: The overlayed output of the modelled biological neuron (cyan) and its equivalent DNN (magenta). Bottom: Inset of the dashed rectangle in the top trace. Extract from Beniaguev et al. 2021
Beniaguev D, Segev I, London M. Single cortical neurons as deep artificial neural networks. Neuron. 2021 Aug 10.
Link to the paper here